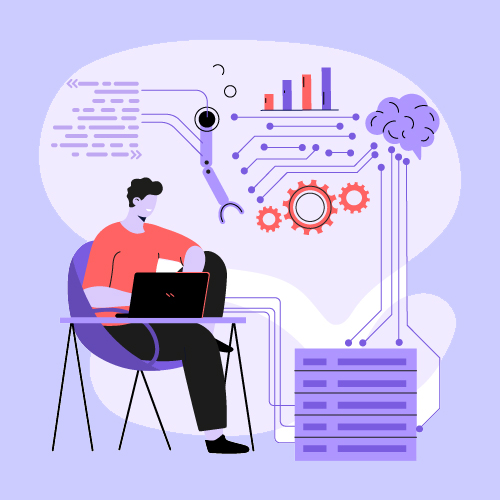
Resource Optimization with Machine Learning
In today’s digital landscape, businesses face a constant challenge: how to maximize cloud performance while keeping costs under control. As cloud environments grow increasingly complex, finding that perfect balance can feel like chasing a moving target. That’s where machine learning comes in-transforming how we approach resource optimization and creating smarter, more efficient systems.
The Resource Optimization Challenge
Managing cloud resources effectively has become a critical business concern. Without proper optimization, companies often face one of two scenarios:
- Over-provisioning: Paying for unused resources “just in case” they’re needed
- Under-provisioning: Saving money but risking performance issues and poor user experience
Traditional manual approaches to resource management simply can’t keep pace with the dynamic nature of modern cloud environments. This is where machine learning shines, offering intelligent solutions that adapt in real-time.
How Machine Learning Transforms Resource Management
Machine learning algorithms are revolutionizing resource optimization by analyzing vast amounts of data and identifying patterns that humans might miss. Here’s how ML is making a difference:
Predictive Resource Scaling
One of the most powerful applications of ML in resource optimization is predictive scaling. Rather than reacting to changes after they occur, machine learning can:
- Analyze historical usage patterns to forecast future demand
- Automatically scale resources up before peak periods
- Scale down during predicted low-usage times
- Maintain optimal performance while avoiding unnecessary costs
This proactive approach ensures your systems have exactly what they need, when they need it-no more, no less.
Intelligent Workload Distribution
Not all workloads are created equal. Machine learning excels at understanding the unique requirements of different applications and distributing resources accordingly:
- Prioritizing critical applications during high-demand periods
- Identifying the most cost-effective instance types for specific workloads
- Balancing loads across different geographic regions to optimize performance
- Suggesting multi-cloud strategies to leverage the best pricing across providers
Cost Analysis and Optimization
Perhaps the most immediate benefit of ML-powered resource optimization is cost reduction. AI-driven cost analysis tools can:
- Identify idle or underutilized resources that can be downsized or eliminated
- Recommend more cost-effective configurations based on actual usage patterns
- Detect anomalies in spending that might indicate inefficiencies
- Continuously monitor cloud spending to keep budgets on track
Real-World Benefits of ML-Powered Optimization
The advantages of implementing machine learning for resource optimization extend beyond just balancing performance and cost:
Significant Cost Savings
Organizations implementing AI-driven resource optimization are seeing impressive results. According to recent data, businesses can reduce cloud expenses by up to 80% through intelligent resource allocation and management.
Enhanced Performance and Reliability
By ensuring resources are available when and where they’re needed most, ML optimization leads to better overall system performance and user experience.
Improved Sustainability
Optimized resource usage isn’t just good for your budget-it’s better for the planet too. By reducing unnecessary computing resources, organizations can significantly decrease their energy consumption and carbon footprint.
Getting Started with ML-Powered Resource Optimization
Ready to leverage machine learning for your resource optimization needs? Here’s how to begin:
- Start with data collection: Ensure you’re gathering comprehensive metrics about your current resource usage and performance
- Choose the right tools: Look for platforms that offer ML-driven insights specifically designed for resource optimization
- Implement gradually: Begin with non-critical workloads to test and refine your approach
- Measure results: Track both cost savings and performance metrics to quantify the impact
- Continuously improve: Machine learning models get better with more data, so maintain a cycle of evaluation and refinement
How DoneDeploy Can Help
At DoneDeploy, we specialize in implementing intelligent resource optimization solutions that leverage the power of machine learning. Our team of cloud infrastructure experts can help you:
- Set up comprehensive monitoring and data collection systems
- Implement ML-powered resource scaling across AWS, GCP, and Azure environments
- Develop custom optimization strategies tailored to your specific workloads
- Create dashboards that provide clear visibility into both performance and costs
With our fixed-price packages and transparent approach, you’ll know exactly what you’re getting and how much you’ll save. Our clients typically see cost reductions of 30-40% while maintaining or improving performance metrics.
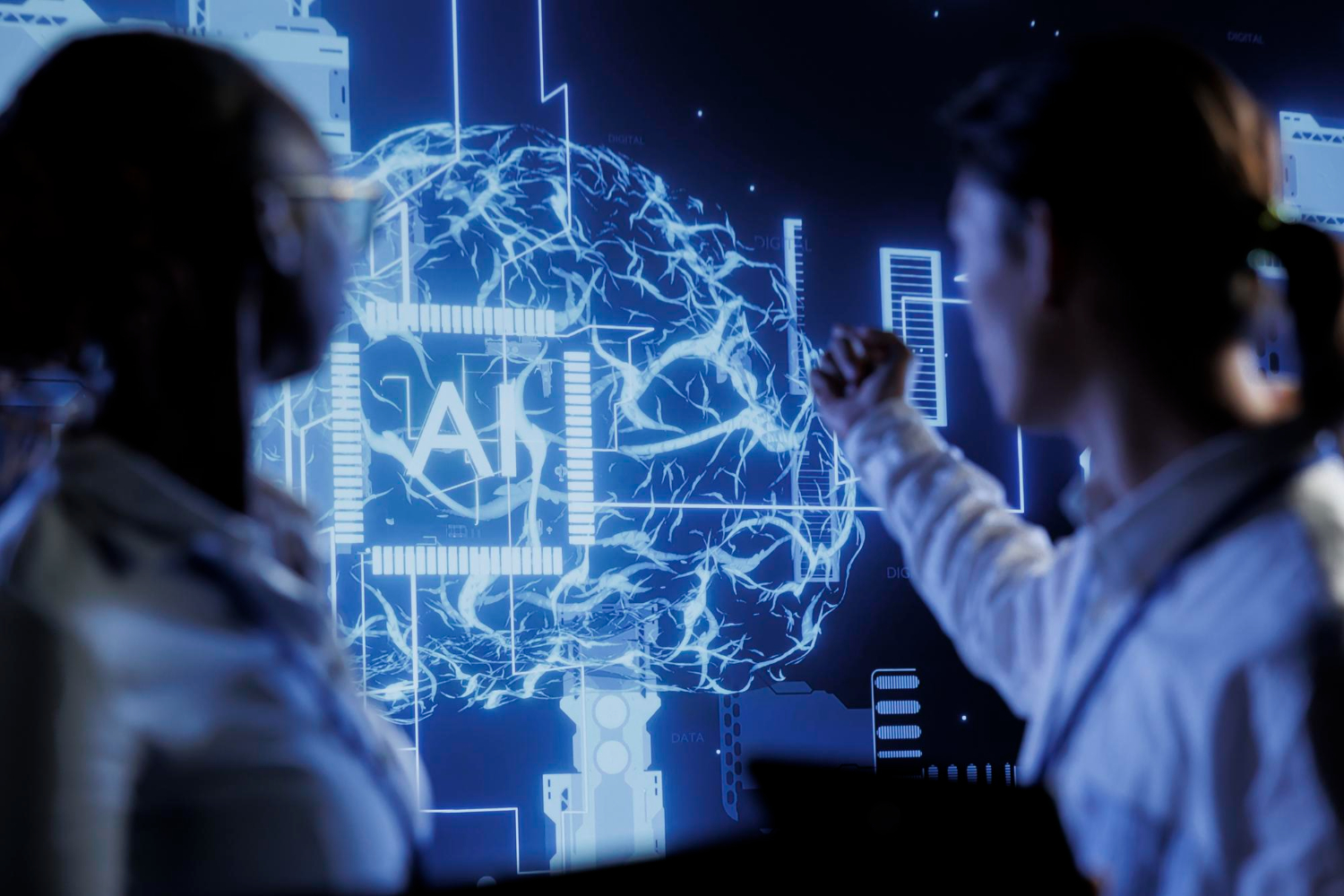
Conclusion
As cloud environments continue to grow in complexity, machine learning offers a powerful solution for balancing performance and cost. By leveraging AI to predict needs, distribute workloads intelligently, and identify optimization opportunities, businesses can achieve significant cost savings while maintaining-or even improving-system performance.
Ready to transform your resource management with machine learning? Visit DoneDeploy.com today to learn how our expert team can help you optimize your cloud infrastructure for both performance and cost.
Share this article
Follow us
A quick overview of the topics covered in this article.
Latest articles
May 16, 2025
May 16, 2025
May 16, 2025